Analyzing monofractal short and very short time series: a comparison of detrended fluctuation analysis and convolutional neural networks as classifiers
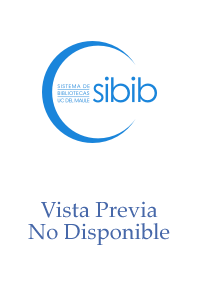
Autor
López, Juan L.
Vásquez-Coronel, José A.
Fecha
2024Resumen
Time series data are a crucial information source for various natural and societal processes. Short time series can exhibit long-range correlations that reveal significant features not easily discernible in longer ones. Such short time series find utility in AI applications for training models to recognize patterns, make predictions, and perform classification tasks. However, traditional methods like DFA fail as classifiers for monofractal short time series, especially when the series are very short. In this study, we evaluate the performance of the traditional DFA method against the CNN-SVM approach of neural networks as classifiers for different monofractal models. We examine their performance as a function of the decreasing length of synthetic samples. The results demonstrate that CNN-SVM achieves superior classification rates compared to DFA. The overall accuracy rate of CNN-SVM ranges between 64% and 98% , whereas DFA’s accuracy rate ranges between 16% and 64% .
Fuente
Fractal and Fractional, 8(8), 460Link de Acceso
Click aquí para ver el documentoIdentificador DOI
doi.org/10.3390/fractalfract8080460Colecciones
La publicación tiene asociados los siguientes ficheros de licencia: