Machine learning and matrix-assisted laser desorption/ionization time-of-flight mass spectra for antimicrobial resistance prediction: a systematic review of recent advancements and future development
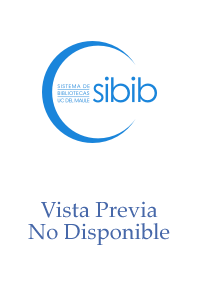
Autor
López-Cortés, Xaviera A.
Manríquez-Troncoso, José M.
Kandalaft-Letelier, John
Fecha
2024Resumen
Background:
The use of matrix-assisted laser desorption/ionization time-of-flight mass spectra (MALDI-TOF MS) combined with machine learning techniques has recently emerged as a method to address the public health crisis of antimicrobial resistance. This systematic review, conducted following Preferred Reporting Items for Systematic reviews and Meta-Analyses (PRISMA) guidelines, aims to evaluate the current state of the art in using machine learning for the detection and classification of antimicrobial resistance from MALDI-TOF mass spectrometry data.
Methods:
A comprehensive review of the literature on machine learning applications for antimicrobial resistance detection was performed using databases such as Web Of Science, Scopus, ScienceDirect, IEEE Xplore, and PubMed. Only original articles in English were included. Studies applying machine learning without using MALDI-TOF mass spectra were excluded.
Results:
Forty studies met the inclusion criteria. Staphylococcus aureus, Klebsiella pneumoniae and Escherichia coli were the most frequently cited bacteria. The antibiotics resistance most studied corresponds to methicillin for S. aureus, cephalosporins for K. pneumoniae, and aminoglycosides for E. coli. Random forest, support vector machine and logistic regression were the most employed algorithms to predict antimicrobial resistance. Additionally, seven studies reported using artificial neural networks. Most studies reported metrics such as accuracy, sensitivity, specificity, and the area under the receiver operating characteristic (AUROC) above 0.80.
Conclusions:
Our study indicates that random forest, support vector machine, and logistic regression are effective for predicting antimicrobial resistance using MALDI-TOF MS data. Recent studies also highlight the potential of deep learning techniques in this area. We recommend further exploration of deep learning and multi-label supervised learning for comprehensive antibiotic resistance prediction in clinical practice.
Fuente
Journal of Chromatography A, 1734, 465262Link de Acceso
Click aquí para ver el documentoIdentificador DOI
doi.org/10.1016/j.chroma.2024.465262Colecciones
La publicación tiene asociados los siguientes ficheros de licencia: