Optimizing hyperparameters in machine learning models for accurate fitness activity classification in school-aged children
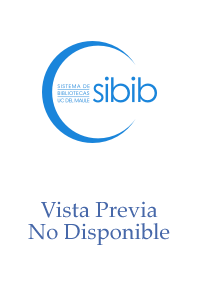
Autor
Calluchi Arocutipa, Britsel
Villegas Cahuana, Magaly
Huanca Hilachoque, Vanessa
Cossio Bolaños, Marco
Fecha
2024Resumen
Classification using machine learning algorithms in physical fitness tests carried out by students in educational centers can help prevent obesity and other related diseases. This research aims to evaluate physical fitness using percentiles of the tests and machine learning algorithms with hyperparameter optimization. The process followed was knowledge discovery in databases (KDD). Data were collected from 1525 students (784 women, 741 men) aged 6 to 17, selected non-probabilistically from five public schools. For the evaluation, anthropometric parameters such as age, weight, height, sitting height, abdominal circumference, relaxed arm circumference, oxygen saturation, resting heart rate, and maximum expiratory flow were considered. Physical Fitness tests included sitting flexibility, kangaroo horizontal jump, and 20-meter fly speed. Within the percentiles observed, we took three cut-off points as a basis for the present research: > P75 (above average), p25 to p75 (average), and < P25 (below average). The following machine learning algorithms were used for classification: Random Forest, Support Vector Machine, Decision tree, Logistic Regression, Naive Bayes, K-nearest neighbor, XGBboost, Neural network, Cat Boost, LGBM, and Gradient Boosting. The algorithms were hyperparameter optimized using GridSearchCV to find the best configurations. In conclusion, the importance of hyperparameter optimization in improving the accuracy of machine learning models is highlighted. Random Forest performs well in classifying the “High” and “Low” categories in most tests but struggles to correctly classify the “Normal” category for both male and female students.
Fuente
International Journal of Advanced Computer Science and Applications, 15(8), 962-972Link de Acceso
Click aquí para ver el documentoIdentificador DOI
doi.org/10.14569/IJACSA.2024.0150895Colecciones
La publicación tiene asociados los siguientes ficheros de licencia: